In the ever-evolving world of machine learning in drug discovery, benchmarking datasets play a pivotal role in driving progress and innovation. They serve as a critical tool for evaluating and comparing different algorithms and models. However, as the field advances, researchers have recognized several key challenges associated with benchmarking datasets.
A blog post from Pat Walters sheds light on many of these challenges, emphasizing the need to address them to ensure the reliability and generalizability of machine learning models. Our observations, detailed in a manuscript where we expand on our machine learning predictions of CYP inhibition, echo his insights on the subject:
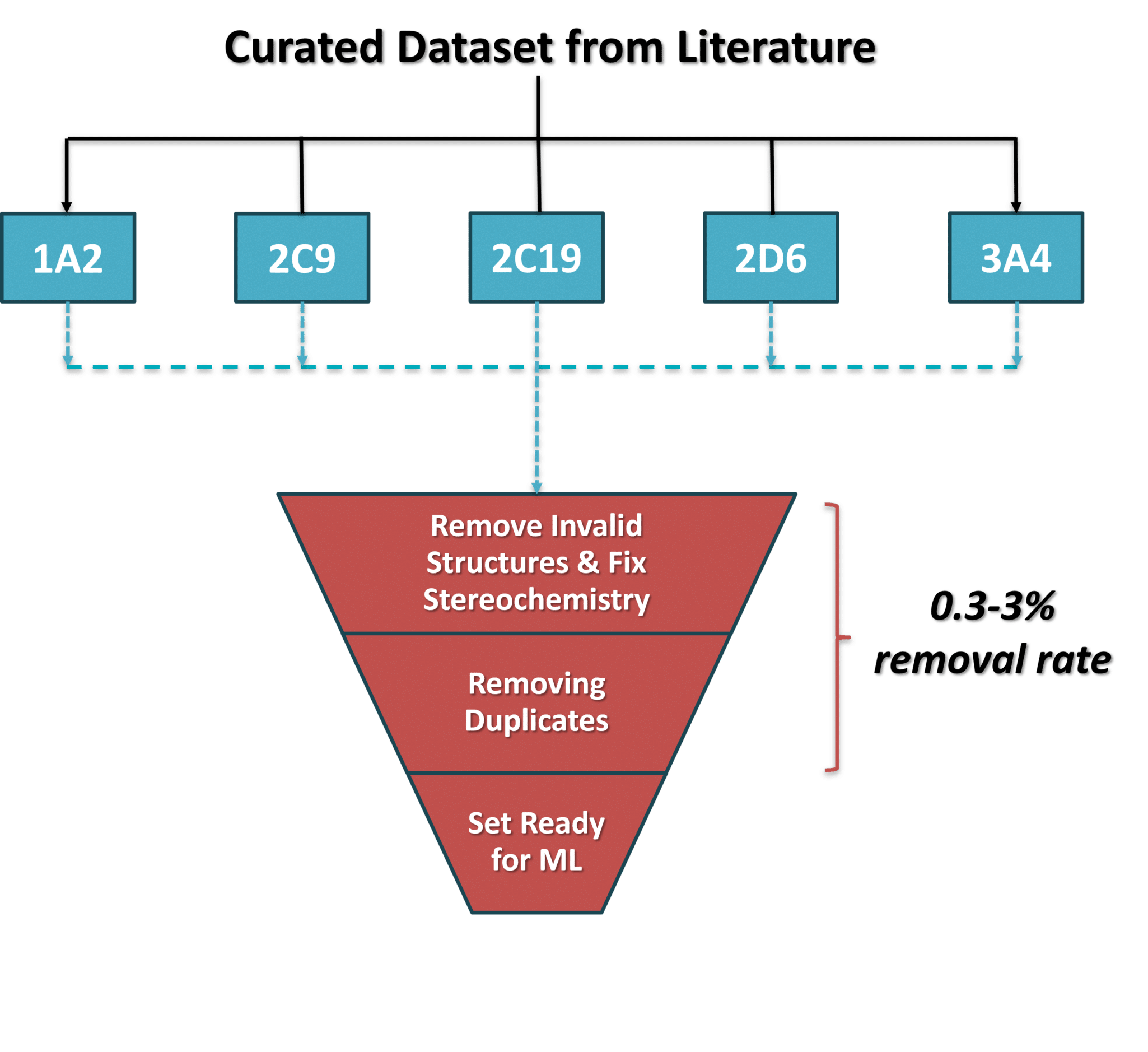
- “consistent chemical representations”. For example, we (and others) have identified multiple instances in which compounds have incorrect protonation or unrealistic tautomeric states, which must be fixed before training a model. Otherwise, these models will follow the well-known computer science adage: garbage-in, garbage-out.
- “data curation errors”, involving the appearance of invalid or duplicate structures in benchmarking datasets. In our work, the available dataset, curated by multiple groups, still contained inconsistencies and invalid or duplicate structures. We went to great lengths to curate these curated sets and remove these structures from our research.
- “stereochemistry”, which we consider to be one of the hardest issues to solve when developing benchmarking sets. As Pat explains in his post, datasets will often contain compounds with undefined chiral centers. This can be the result of curation errors, or of genuine uncertainty as to the proper definition of stereochemistry. Of course, biologically active compounds have very well-defined chiral centers (see for example R/S-warfarin, R/S-thalidomide, and others), and thus it is important to not randomly assign stereochemistry.
You can also check out some of our other work where these issues continually need special attention.